The unlikely but fruitful waltz of a curious couple: Phenotypic plasticity and learning theory
by Miguel Brun-Usan
6 August 2018
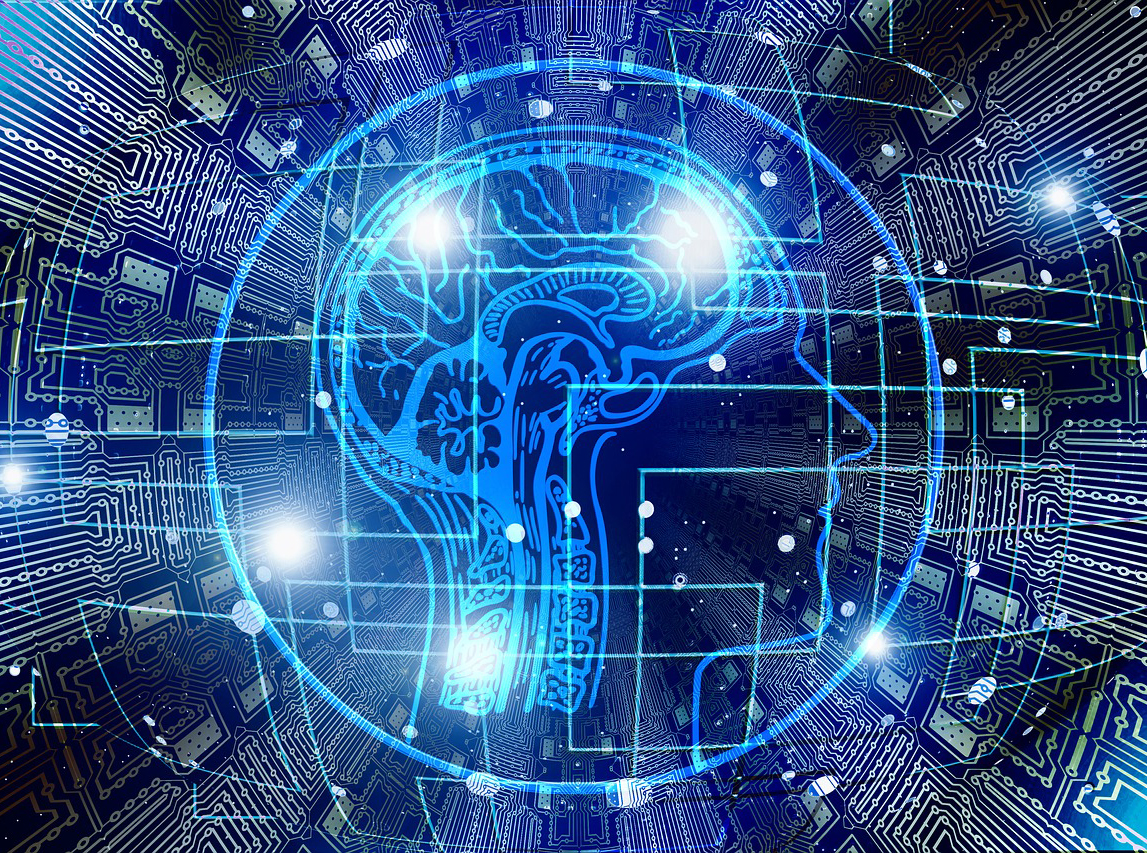
When the Modern Synthesis was put together in the early decades of the twentieth century, natural selection became the obvious focus of evolutionary thought. Although this focus has increased our understanding of biological evolution by orders of magnitude, it tells us little about how phenotypic variation is produced. Variation – the third evolutionary pillar envisioned by Darwin (the other two being selection and heredity) – is typically taken for granted, and assumed to follow from the random, isotropic, and unbiased genetic changes that arise by mutation.1
The lack of a theory of variation has been acknowledged ever since the 19th century, but it became increasingly pressing in the latter half of the 20th century, as groups of researchers became concerned that the Modern Synthesis turned evolution into a theory of genes, rather than morphologies or phenotypes. Genetic theory, for all it’s worth, does not appear to explain how the physical features of organisms are produced, or how such phenotypes can sense and respond to the environment.2,3 This is a major issue, considering that phenotypes are the only thing that natural selection can directly ‘see’ or act upon.
What is, then, responsible for bringing phenotypes into being? The answer is development. Evolutionary phenomena such as robustness, evolvability, phenotypic plasticity, and so on are ultimately properties of the developing system, not the genotype alone. By understanding how development works at a mechanistic level, we may be able to reveal the causal relationships between these phenomena, the processes that produce phenotypic variation, and natural selection.4 In more recent years, the integrative framework of the extended evolutionary synthesis (EES) seeks to build a coherent and unifying conceptual framework in which the evolutionary role of developmental processes can be addressed.5
One of the most prominent features of organisms, whose evolutionary role was difficult to discern in the Modern Synthesis but has become a key topic within the EES, is phenotypic plasticity (the sensitivity of the phenotype to environmental factors). If phenotypes are assumed to be genetically encoded, phenotypic plasticity does not appear to deserve any special attention, and thus requires no further explanation. However, the only way for the environment to alter phenotypes is by affecting development, a process that is only partially determined by genes.6
Phenotypic plasticity functions at multiple biological levels. At the organismal level, plasticity is often considered an adaptive feature because it allows organisms to respond faster to environmental changes compared to responses that rely on selection on genetic variation. At the cellular level, phenotypic plasticity is required to build up a complex and functional multicellular organism.7 Since all cells within a multicellular organism are genetically identical, cell differentiation relies on cell plasticity. In addition, cell plasticity is also involved in the process of cell de-differentiation, a crucial event for wound healing and regeneration.
Cell plasticity can be described as the mathematical function that links one environmental factor (input) with a specific cell state (output). In many systems, this approach results in so-called reaction norms: representations of phenotypes (e.g., trait values) as a simple (e.g., linear or monotonic) function of one environmental input. However, a closer look at the mechanics of cell differentiation reveals that cells use a large number of signaling cascades and juxtaposed mechanisms (e.g. mechanotransduction, internal biochemical clocks, etc) to gather information about when, where, and how to differentiate.
How do cells manage to integrate such a complex biochemical environment into a coherent and orchestrated response? The answer is through environmentally-sensitive regularly networks, including gene-regulatory networks (GRNs). Some elements of GRNs are affected by chemicals coming from outside the cell, thus ‘sensing’ the environmental conditions. So far, so good. But … how many signals can a GRN process? Do they ignore some signals while focusing on others? Among the signals that matter, how are these combined to generate a meaningful phenotypic response? What is the underlying logic of the process?
To answer these questions, one ought to abandon unidimensional reaction norms and search for mathematical functions that, given an arbitrary number of input parameters (environmental factors), generate a single output (cell phenotypic state). Fortunately, these functions do exist, and have been known for many years, especially in the field of computer sciences: they are the logical functions derived from Boolean algebra. These functions allow us to describe specific relationships between combinations of environmental cues (inputs) and the phenotypic responses (outputs). Thinking of development in this way allows us to apply principles derived from computer science and learning theory to understand multi-dimensional cell plasticity. The EES framework helps to build conceptual bridges between these disciplines and evolutionary biology.
In learning theory, there are two types of algorithms. In generative algorithms, the system has to generate an adequate response in the form of an adaptive variant. In discriminatory algorithms, the system has to classify a series of elements, stating whether they belong to some category or not. In our research group at the University of Southampton we realized that the evolution of cell plasticity is formally equivalent to a simple categorization learning process familiar in the context of neural networks: what cells ultimately do is to classify different combinations of environmental inputs (e.g. morphogen gradients) into a small set of possible categories (cell states). Exploring both types of algorithm can be useful to understand how plasticity evolves, and how it shapes the capacity for future evolution.
Previous research has shown that, under some circumstances, evolving systems are able to use information gathered during their evolutionary past to generate an adaptive response in novel and previously unseen environments.8,9 Genotype-phenotype-maps (GPMs) describe the relationship between genotypes and phenotypes. When organisms evolve in fluctuating environments over long periods, they acquire a specific genotype-phenotype-map (GPM) where the phenotypes that are fitter in all these past environments are more likely to arise under (non-directed) mutation than less fit phenotypes. Since GPMs have an inherent structure, or variational properties,3,10 it sometimes happens that correlated phenotypes lie close to one another in the GPM.
Let us now place this organism in an environment that, despite being new, exhibits some similarities with previously experienced environments. The logical consequence of having correlated phenotypes in the GPM is that the evolving system will be more likely to produce phenotypes with high fitness in this new environment. In other words, the organism has used past experiences of its lineage to offer the right response to a new challenge, a hallmark of learning. Whilst it seems intuitive that simple forms of learning may be commonplace (e.g. extrapolation, where a trait X that is fitter in environments with high concentrations of the factor Y becomes even larger when the system evolves in a new environment with even more Y), the existence of more sophisticated forms of learning (e.g. generalization, which involves “storing” high-level correlations between different traits and environmental variables) is clearly not trivial. It deserves more attention.
In the generative case, the learning capabilities of plastic cells require the existence of specific ‘rules’ that connect environment and phenotype; a relationship which may be called the environment-phenotype-map (EPM). Unfortunately, the EPMs underlying phenotypic plasticity are much less studied than the GPMs despite the environment being an indispensable source of matter, energy, and information in the process of development. Whilst the tenet “there cannot be phenotypes without development”10 is true, it is equally true that “there cannot be phenotypes without environment”.
In our research group, we are trying to disentangle how multi-dimensional environments direct the determination of cellular phenotypes. That is, we aim to better understand how different EPMs emerge from network dynamics, what kind of logical functions, plastic responses and learning capabilities they can perform, and how these features are linked to specific network topologies and properties.
In order to address these questions, we used a computational model of non-symmetric GRNs with continuous (non-Boolean) unsigned state variables and explicit environmental factors. Overall, our results revealed a set of structural, dynamical, and functional commonalities between GRNs and neural networks. These commonalities allow us to transfer knowledge derived from learning theory to understand cell plasticity. This is possible because, in simple terms, gene networks might have evolved according to the same principles by which cognitive systems learn.
Our ultimate goal is to assess how these learning capabilities were involved in the evolutionary transition to multicellularity.11 In this transition, which happened at least 40 times in the history of life on Earth, single multi-stable (plastic) cells were entangled in a new whole; a new higher-level of complexity.12 The functional integration of the new level of complexity crucially depends on the ability of the lower-level entities to correctly interpret and respond to a vastly rich set of inter-cellular signals. Coming from free-living states in which the environmental dimensionality was relatively low, the transition to a multi-cellular state might have been very challenging. If these single cells could undergo proper learning, their ability to face the new hyper-complex environment would be greatly improved, and the likelihood of a successful transition might consequently increase.
The very existence of the multicellular neural networks in our brains, which allow us to think about evolution, may depend on the basic learning capabilities exhibited by some plastic single-celled microorganisms which dwelt in the waters of ancient ponds.
This work is part of project #3 in the EES research program, entitled How evolution learns from experience, under the theme Evolutionary Innovations.
1. Gilbert SF. 2003. Int J Dev Biol 47:467-477. 2. Waddington CH. 1957. Allen & Unwin. 3. Alberch P. 1983. Evolution 37:861-863. 4. Salazar-Ciudad I. 2007. Int Comp Biol 47:390-400. 5. Laland KN, et al. 2015. Proc R Soc B 282:20151019. 6. Newman SA, Müller GB. 2000. J Exp Zool 288:304-317. 7. Sebé-Pedrós A, Degnan BM, Ruiz-Trillo I. 2017. Nat Rev Gen 18:498-512. 8. Watson RA, Wagner GP, Pavlicev M, Weinreich DM, Mills R. 2014. Evolution 68:1124-1138. 9. Kouvaris K, Clune J, Kounios L, Brede M, Watson RA. 2017. PLoS Comp Biol 13:e1005358. 10. Salazar‐Ciudad I. 2006. J Exp Zool (Mol Dev Evol) 306:107-125. 11. Szathmáry E, Maynard Smith J. 1995. Nature 374:227-232. 12. Grosberg RK, Strathmann RR. 2007. Annu Rev Ecol Evol Syst 38:621-654.